In an era defined by rapid technological advancement and exponentially growing data, organizations are seeking ways to turn raw data into actionable insights more efficiently than ever. Traditional Business Intelligence (BI) platforms, while powerful, often fall short of meeting the high-speed demands of modern business. Enter Augmented Analytics, a breakthrough that is transforming how companies make decisions by streamlining data discovery, analysis, and interpretation through the power of Artificial Intelligence (AI) and Machine Learning (ML).
What is Augmented Analytics?
Augmented Analytics refers to the use of enabling technologies like machine learning, natural language processing (NLP), and AI to enhance data analytics practices. Rather than requiring data scientists to do all the heavy lifting, augmented analytics tools help to automate data preparation, insight generation, and data visualization. The goal is simple but powerful: to make data insights more accessible to professionals across all levels of a business — not just analysts.
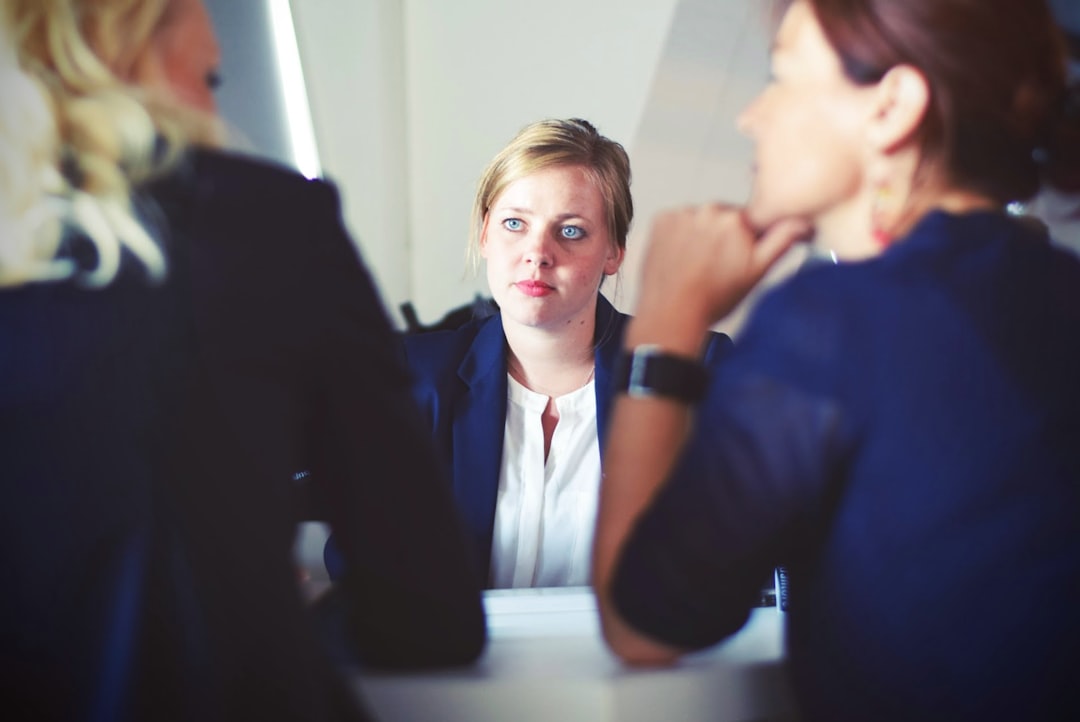
Bridging the Gap Between Data and Decisions
In traditional BI systems, decision-makers often rely on reports prepared by data analysts. This back-and-forth can slow down critical business decisions. Augmented analytics tools, by contrast, allow business users to ask questions in natural language and receive instant insights generated through AI algorithms. This automation enables a near real-time shift from data to decision.
For example, a regional sales manager can quickly inquire, “Why did sales drop in the northeast last quarter?” The system can analyze historical sales data, external market factors, and internal performance metrics to generate a contextual, actionable explanation — complete with visualizations and predictive suggestions.
Core Benefits of Augmented Analytics in BI
There are several reasons why augmented analytics is reshaping the future of BI:
- Speed and Efficiency: Automating tasks like data cleansing, aggregation, and statistical modeling reduces the time needed to uncover insights.
- Democratized Data Access: Non-technical users gain the ability to interact directly with data, eliminating the bottleneck of relying solely on analysts.
- Enhanced Predictive Analysis: AI models identify trends and patterns faster, helping businesses anticipate outcomes before they occur.
- Reduced Human Error: Automation minimizes the risk of biased or incorrect interpretations that can skew decisions.
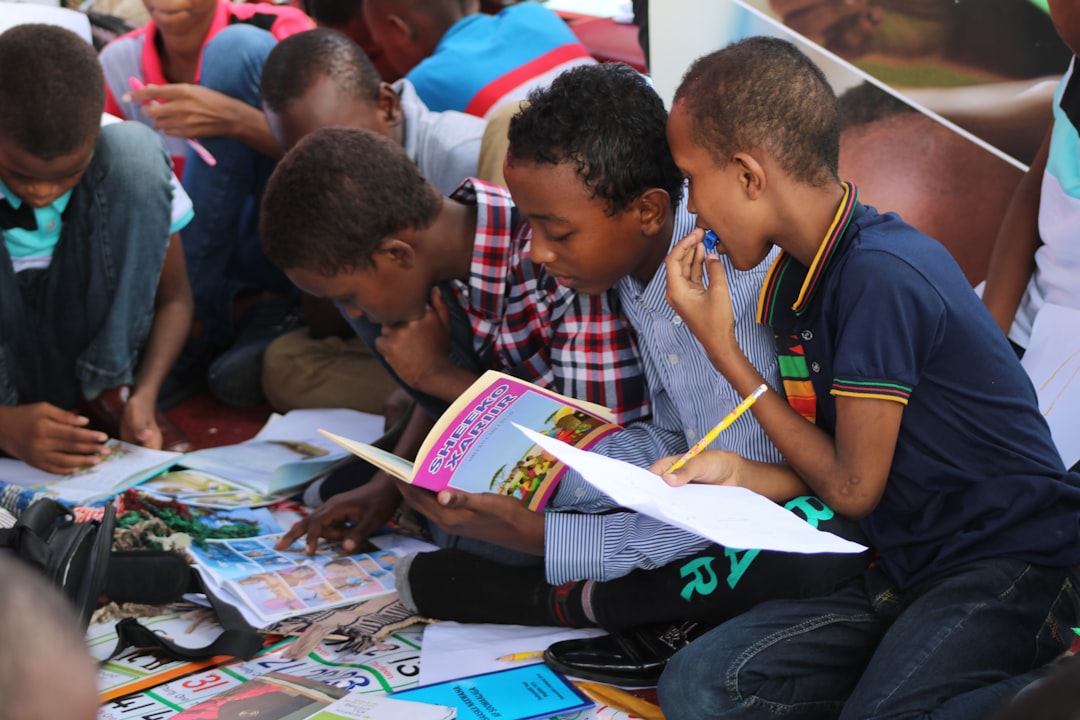
Applications Across Industries
From retail to healthcare, augmented analytics is finding applications across sectors. In finance, it helps detect fraudulent transactions in real time. In manufacturing, it can forecast production inefficiencies and recommend optimization strategies. Healthcare institutions use it to predict patient outcomes and personalize care approaches based on data-driven patterns.
Retailers leverage augmented analytics to understand customer behavior, optimize inventory, and fine-tune marketing strategies. Meanwhile, HR departments in large organizations apply it to evaluate employee performance trends and improve retention strategies. The adaptability and scalability of these tools make them critical in any industry striving for data-informed agility.
Challenges and Considerations
While the promise of augmented analytics is substantial, there are challenges to keep in mind. Data quality still plays a foundational role; flawed or incomplete data can undermine even the smartest algorithms. Furthermore, businesses must ensure that users understand how to interpret AI-generated insights, avoiding blind trust in machine output.
Organizational change management is also crucial. Employees need to embrace a shift toward data-driven decision making, often requiring investment in training and cultural adaptation. Additionally, issues related to data governance, privacy, and compliance need to be addressed — especially when dealing with sensitive information or operating in regulated industries.
The Future of Business Intelligence
As the volume and complexity of data continue to grow, augmented analytics will become less of a luxury and more of a necessity. By embedding intelligence throughout the analytics process, augmented analytics stands poised to redefine how businesses think, act, and compete. The fusion of human intuition and machine precision is opening doors to faster, smarter, and more confident decision-making — a true transformation from data overload to data empowerment.
Frequently Asked Questions
-
What distinguishes augmented analytics from traditional BI?
Augmented analytics employs AI and machine learning to automate data analysis tasks, enabling faster insights and broader accessibility compared to traditional methods that typically require manual input and interpretation. -
Do users need coding skills to use augmented analytics tools?
No, many platforms are designed with user-friendly, no-code interfaces that allow even non-technical users to perform complex data analysis and generate visual insights using natural language queries. -
Is augmented analytics suitable for small businesses?
Yes, many scalable augmented analytics solutions are designed to cater to businesses of all sizes, offering affordable plans and intuitive tools that do not require dedicated data science teams. -
How does augmented analytics handle data privacy?
Reputable platforms incorporate data governance protocols and comply with industry regulations such as GDPR and HIPAA to protect user data and ensure ethical data use.